Stay updated on news, articles and information for the rail industry
February 2018
Rail News: MOW
MOW: Big Data in railroading moves from theory to practice
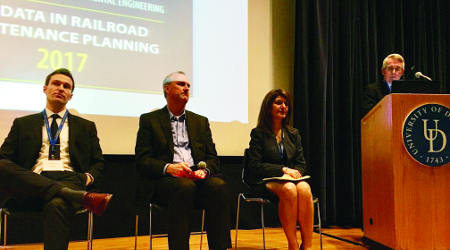
By Daniel Niepow, Associate Editor
It was a sunny mid-December day as academics, railroaders and data scientists filed into a basement theater on the University of Delaware’s campus.
The group — nearly 200 people in all — assembled for the university’s fourth annual “Big Data in Railroad Maintenance Planning” conference in Newark, Delaware. After attendees took their seats, University President Dennis Assanis took the stage to tout the wide-reaching potential of data analytics.
“Data science can advance human understanding in all branches of science and the humanities,” he said. “It can help us address the grand challenges we face in society.”
Over the next two days, a range of presenters showed just how data science can tackle some of the rail industry’s “grand challenges.” Many speakers focused on using railroads’ ever-expanding collection of track data to drive safety and cut costs, but the opportunities to leverage Big Data abound in nearly every corner of the industry — whether that involves managing locomotive health, improving fuel efficiency or boosting customer service.
“We’re getting past the realm of ‘this is how we should be using Big Data’ to the realm of ‘this is how we are using it,’” said Allan Zarembski, research professor and director of the University of Delaware’s Railroad Engineering and Safety Program. “There’s a lot more to go, and we hope to continue to improve.”
For Union Pacific Railroad, one Big Data use case dates back more than a decade. During one of the morning sessions, UP Senior Vice President and Chief Information Officer Lynden Tennison highlighted the Class I’s wayside detection system, which was implemented in 2002.
Patterns predict failures
The railroad has applied a pattern-matching algorithm to sort the data collected through that system and help identify potential equipment failures. UP has significantly reduced the number of bearing-caused wheel failures by leveraging this data, Tennison said.
In addition, UP continues using Machine Vision technology to keep tabs on its rolling stock. The portal over track can take thousands of images per second as trains roll by. The goal: to use machine learning to detect mechanical problems.
“Eventually, we can start taking the person out of the loop for decision-making,” Tennison said.
Time for a quick break before the morning session continues #BigDataInRail pic.twitter.com/1iexQ0E0KQ
— Progressive Railroad (@rail_pro_mag) December 14, 2017
Still, getting to that point requires finding more ways to sort through seemingly endless gobs of data. During another presentation, BNSF Railway Co. Assistant Director of Roadway Planning Michael Messner noted his railroad’s amount of data has grown “exponentially” over the past four or five years. As of late 2017, the Class I logged data for 270,000 track miles.
At any given time, BNSF has five geometry cars actively collecting track data across its network. Messner’s talk focused on using geometry car data to create what he termed a “comprehensive surfacing plan.”
“Now we have the data to show that we can be much more on the proactive side of the equation,” Messner said.
But as opportunities grow in the Big Data universe, so does the potential for making errors. David Staplin, Amtrak’s retired deputy chief engineer, warned attendees against allowing Big Data to lead to “big mistakes.”
“We need to be careful in the march forward in Big Data … Failure to do so can be catastrophic,” he said.
Avoiding pitfalls
As an example, Staplin cited American Airlines’ scheduling hiccups for holiday flights. A glitch in the company’s scheduling system gave too many pilots time off, which would have affected thousands of flights. The airline and its pilots eventually hashed out a deal to staff the flights, but the scenario underscored the potential for unintended consequences in rolling out new technology.
“Even though they claim to have solved the problem, it represents a big erosion in confidence in a business enterprise that’s basic to our economy,” said Staplin, who serves as chairman of the University of Delaware’s Railroad Advisory Board.
Risks notwithstanding, there are tangible benefits to employing new technologies and leveraging data streams. Case in point: Amtrak’s record $3.2 billion revenue in fiscal-year 2017. The national passenger railroad used data analytics to more accurately measure the effectiveness of its various marketing campaigns, which helped drive revenue growth, said Ghada Ijam, Amtrak’s former chief information officer.
“As a result of that, the marketing department was able to gain more ridership and gain more revenue,” Ijam said, adding that the record revenue growth followed a “significant decline” in revenue in the prior year.
Still, Amtrak’s No. 1 priority in the use of data analytics is to drive safety, said Wick Moorman, who retired as Amtrak’s Chief Executive Officer Dec. 31. Moorman delivered a keynote address at the conference.
Improving passenger experience
As the price of new and emerging technologies continues to drop — costs have been “asymptotically approaching zero for a long time,” he noted — across-the-board improvements become more attainable, too. Along with safety, the overall customer experience can be enhanced with data analytics.
“We’re behind the airlines in this category,” Moorman said. “Big Data can help us make huge improvements in the Amtrak customer experience. It’s a significant benefit for all of us.”
As with the marketing improvements, Amtrak can use data to gauge customers’ opinions on their experience and find areas for improvement.
And, to be sure, there’s plenty of room for improvement in Big Data-related technology itself, as Zarembski and other presenters pointed out. Especially in the realm of machine learning, railroaders must be patient as the technology corrects itself over time.
“It’s like a kid learning to talk. For the first three years they just blabber. We think it’s cute, but when the machine blabbers, people get frustrated,” said Dhaivat Parikh, senior managing consultant of strategy and analytics at IBM Global Business Services. “But the point is that you have to be patient with this.”
For its part, IBM has developed algorithms to align and sort through machine-generated data from geometry cars, said John Briscoe, a consultant with the company.
For Zarembski, the conference’s growing popularity underscores the rail industry’s increasing interest in data analytics. The 2017 event attracted Class I railroaders, transit leaders and suppliers from around the globe.
Even at the first Big Data in rail conference in 2014, attendance far surpassed initial expectations, leading the university to move the event to a larger venue.
Amtrak's Michael Craft discusses how to share track geometry data in different ways for people who need it. #BigDataInRail pic.twitter.com/LYtuZFPGzK
— Progressive Railroad (@rail_pro_mag) December 14, 2017
Not a means to an end
Next month, the university will hold a two-day “short course” to teach railroaders how to perform the underlying calculations needed to leverage Big Data. Zarembski decided to offer the course at the request of previous conference attendees.
He hopes future conferences will include more rolling stock applications in the Big Data realm.
For now, railroads and their suppliers proceed with caution into the growing pool of data. That was reflected in Moorman’s keynote address, which ended with a final caveat for attendees.
“This is good stuff from a business standpoint,” he said. “It allows us to do things we’ve never done before, but it’s not in itself a means to an end.”
That was a shared sentiment among the other presenters, including Grant Engel, the Southeastern Pennsylvania Transportation Authority’s insights and analytics manager. He was one of three panelists participating a question-and-answer session moderated by Moorman.
“Collecting Big Data for the sake of Big Data needs to be guarded against,” Engel said, adding that it’s important to ensure “Big Data doesn’t become big siloed data.”
Email questions or comments to daniel.niepow@tradepress.com.